Bias in Big Data and the Implications for Local Governments
The views expressed are those of the author and do not necessarily reflect the views of ASPA as an organization.
By Matthew Teal
June 12, 2019
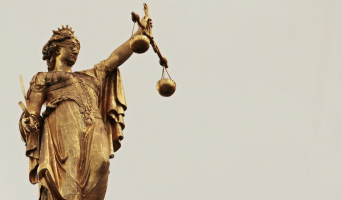
The final column in this series will look at the risk of bias in artificial intelligence (AI) and Big Data, implications of that bias for local governments and practical steps local governments can take to counter the bias. Modern societies have turned over increasing amounts of decision-making to complex algorithms, often with the argument that the resulting decisions must be valid because, “The data does not lie.” This view of AI and Big Data is fundamentally flawed and even dangerous. From a local government perspective, this bias shows itself most prominently in hiring decisions and the criminal justice system.
According to mathematician Kathy O’Neil’s book Weapons of Math Destruction: How Big Data Increases Inequality and Threatens Democracy, the push for using Big Data and AI algorithms in every sector of American life exploded after the Great Recession. This push occurred despite evidence many of the same algorithms played a key role in the Great Recession itself: “[T]he housing crisis, the collapse of major financial institutions, the rise of unemployment—all had been aided and abetted by mathematicians wielding magic formulas.”
In a way, this post-Great Recession expansion was logical. When the economy was on the brink of ruin, the way businesses and governments could keep from drowning was by squeezing every bit of cost they could out of their operations. As O’Neil puts it:
“Algorithms promised spectacular gains. A computer program could speed through thousands of resumes or loan applications in a second or two and sort them into neat lists, with the most promising candidates on top. This not only saved time but also was marketed as fair and objective. After all, it didn’t involve prejudiced humans digging through reams of paper, just machines processing cold numbers.”
These algorithms were treated as black boxes, meaning that humans could see the input data and the output results but have no way of understanding what the model did to achieve those results. This lack of transparency, appearance of impartiality and inability to appeal an unfavorable result had serious implications. O’Neil writes that the algorithm’s, “Verdicts, even when wrong or harmful, were beyond dispute or appeal. And they tended to punish the poor and the oppressed in our society, while making the rich richer.”
However, businesses and governments have gradually come to realize that many algorithms are deeply biased, particularly against women and individuals of color. In a March 1, 2019 article for The Wall Street Journal, John Murawski notes that:
“Microsoft Corp. and Salesforce.com Inc. have already hired ethicists to vet data-sorting AI algorithms for racial bias, gender bias and other unintended consequences…Microsoft and Google parent Alphabet Inc. have started disclosing the risks inherent to AI in their Securities and Exchange filings, a move that some believe presages a standard corporate practice.”
From a local government perspective, this bias has and will continue to cause legal and social risks. Hiring platforms that allow candidates to upload their resumes and cover letters frequently use AI-powered algorithms as a first cut to determine which resumes get reviewed by an actual human. Bias in these algorithms could result in less diverse applicant pools for hiring managers to invite for interviews. In the criminal justice realm, sentencing guidelines and predictive policing models are both powered by AI models. As Karen Hao noted in a January 21, 2019 article for MIT Technology Review, police departments are strapped for resources and are using so-called “risk assessment algorithms” that treat correlations in historical arrest data the same as causations to predict where to allocate police resources. Hao argues that,
“Populations that have historically been disproportionately targeted by law enforcement—especially low-income and minority communities—are at risk of being slapped with high recidivism scores. As a result, the algorithm could amplify and perpetuate embedded biases and generate even more bias-tainted data to feed a vicious cycle. Because most risk assessment algorithms are proprietary, it’s also impossible to interrogate their decisions or hold them accountable.”
How can local governments manage the risk of bias in AI and Big Data? Simply being aware of the problem is a critical first step. Second, before signing a contract agreeing to use a company’s algorithms, local governments should demand that those algorithms be transparent, rather than black boxes. Third, local governments can also partner with third-party organizations that will audit the algorithms and the underlying data to check for evidence of bias. Finally, there may be certain areas of local government operations where it may make more sense to deliberately not use AI and Big Data. For example, a January 25, 2019 article by James Vincent for The Verge and a April 19, 2019 article by Sigal Samuel for Vox demonstrate the ongoing and evolving debate around gender and racial bias in facial recognition technology.
Bias in AI and Big Data is a complicated and fast-moving topic of debate among mathematicians, computer scientists, civil libertarians and other experts. Any local government looking to use AI and Big Data, particularly for hiring and criminal justice-related issues, should approach the topic with eyes wide open.
My opinions are my own and do not represent the University of North Carolina at Chapel Hill.
Author:
Matthew Teal, MA, MPA
Policy Analyst
University of North Carolina at Chapel Hill
Email: [email protected]
Twitter: @mwteal




(5 votes, average: 3.40 out of 5)
Loading...
Bias in Big Data and the Implications for Local Governments
The views expressed are those of the author and do not necessarily reflect the views of ASPA as an organization.
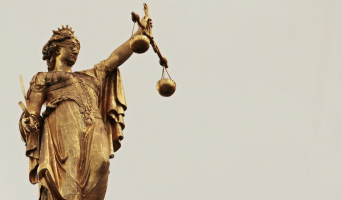
By Matthew Teal
June 12, 2019
The final column in this series will look at the risk of bias in artificial intelligence (AI) and Big Data, implications of that bias for local governments and practical steps local governments can take to counter the bias. Modern societies have turned over increasing amounts of decision-making to complex algorithms, often with the argument that the resulting decisions must be valid because, “The data does not lie.” This view of AI and Big Data is fundamentally flawed and even dangerous. From a local government perspective, this bias shows itself most prominently in hiring decisions and the criminal justice system.
According to mathematician Kathy O’Neil’s book Weapons of Math Destruction: How Big Data Increases Inequality and Threatens Democracy, the push for using Big Data and AI algorithms in every sector of American life exploded after the Great Recession. This push occurred despite evidence many of the same algorithms played a key role in the Great Recession itself: “[T]he housing crisis, the collapse of major financial institutions, the rise of unemployment—all had been aided and abetted by mathematicians wielding magic formulas.”
In a way, this post-Great Recession expansion was logical. When the economy was on the brink of ruin, the way businesses and governments could keep from drowning was by squeezing every bit of cost they could out of their operations. As O’Neil puts it:
These algorithms were treated as black boxes, meaning that humans could see the input data and the output results but have no way of understanding what the model did to achieve those results. This lack of transparency, appearance of impartiality and inability to appeal an unfavorable result had serious implications. O’Neil writes that the algorithm’s, “Verdicts, even when wrong or harmful, were beyond dispute or appeal. And they tended to punish the poor and the oppressed in our society, while making the rich richer.”
However, businesses and governments have gradually come to realize that many algorithms are deeply biased, particularly against women and individuals of color. In a March 1, 2019 article for The Wall Street Journal, John Murawski notes that:
From a local government perspective, this bias has and will continue to cause legal and social risks. Hiring platforms that allow candidates to upload their resumes and cover letters frequently use AI-powered algorithms as a first cut to determine which resumes get reviewed by an actual human. Bias in these algorithms could result in less diverse applicant pools for hiring managers to invite for interviews. In the criminal justice realm, sentencing guidelines and predictive policing models are both powered by AI models. As Karen Hao noted in a January 21, 2019 article for MIT Technology Review, police departments are strapped for resources and are using so-called “risk assessment algorithms” that treat correlations in historical arrest data the same as causations to predict where to allocate police resources. Hao argues that,
How can local governments manage the risk of bias in AI and Big Data? Simply being aware of the problem is a critical first step. Second, before signing a contract agreeing to use a company’s algorithms, local governments should demand that those algorithms be transparent, rather than black boxes. Third, local governments can also partner with third-party organizations that will audit the algorithms and the underlying data to check for evidence of bias. Finally, there may be certain areas of local government operations where it may make more sense to deliberately not use AI and Big Data. For example, a January 25, 2019 article by James Vincent for The Verge and a April 19, 2019 article by Sigal Samuel for Vox demonstrate the ongoing and evolving debate around gender and racial bias in facial recognition technology.
Bias in AI and Big Data is a complicated and fast-moving topic of debate among mathematicians, computer scientists, civil libertarians and other experts. Any local government looking to use AI and Big Data, particularly for hiring and criminal justice-related issues, should approach the topic with eyes wide open.
My opinions are my own and do not represent the University of North Carolina at Chapel Hill.
Author:
Matthew Teal, MA, MPA
Policy Analyst
University of North Carolina at Chapel Hill
Email: [email protected]
Twitter: @mwteal
Follow Us!