Thinking Clearly About Causality
The views expressed are those of the author and do not necessarily reflect the views of ASPA as an organization.
By Nathan Favero
October 27, 2019
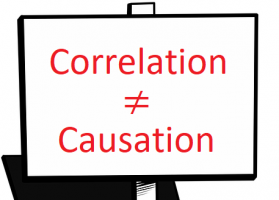
Even with the benefit of hindsight, it can be hard to sort out the precise effects of a decision.
Maybe I notice an uptick in employee productivity following a goal-setting workshop. But was the uptick really caused by the workshop? Or did the addition of a new colleague around the same time boost everyone’s dedication?
The topic of causality is a natural follow-up to my last column on evidence-based management. After all, social scientists are increasingly focused on trying to improve our understanding of causal relationships as we go about assembling evidence.
My favorite tool for thinking about causality is a simple framework that can be used with any two variables. To illustrate, let’s use the example of a professional development program and its effect on job satisfaction.
Suppose I notice that employees who participate in the program are more satisfied with their jobs. In other words, participation is correlated with satisfaction. There are five different reasons we might find this correlation:
Possibility 1: Reverse-causality. My two variables might be correlated because satisfaction causes participation in the program. Satisfaction with the job may drive people to do more, leading them to sign up for the professional development program. If so, I would likely find that those who had participated in the program were happiest with their jobs, even if the program itself had no effect on job satisfaction.
Possibility 2: A confounding factor. Some third variable (a confounding factor) may drive the correlation between participation and satisfaction. For example, suppose that workloads of employees vary widely, with some employees feeling overworked to the point of frustration. Perhaps only those with a manageable workload (who also enjoy their jobs) feel that they can afford the time required to participate in the professional development program. In this case, employee workload is a key driver of both job satisfaction and participation in the program, causing satisfaction and participation to be correlated with one another.
Possibility 3: Coincidence. The world is full of seemingly-random coincidences, so a non-zero correlation can arise without any real reason or explanation. This problem occurs most often with small samples or with small correlations. If only two employees participated in the professional development program, I shouldn’t read too much into the fact that they’re both especially happy with their jobs. With such a small sample, it could be a coincidence. This concern is linked to the concept of, “Statistical insignificance,” which helps to weed out results based on small samples, when such coincidences are likely.
Possibility 4: Research design problems. This is a somewhat broad category of possibilities but includes things like measurement error or people dropping out of your sample. Suppose that the people who participate in the professional development program are told by the program directors that they’ll later be surveyed, and that the directors will look bad unless participants say they now love their jobs. Results of future attempts to measure job satisfaction may now be contaminated if program participants don’t want to disappoint the program directors. Or suppose that participation in the program helps to clarify for employees whether they are a good fit for the organization, and thus accelerates leaving the organization for employees who are unhappy. Even if the program didn’t alter satisfaction, a survey of program alumni might find only happy employees since the others have already left.
Possibility 5: Causality. If you can rule out the prior four possibilities, then you can pretty safely conclude that participation in the program did indeed cause higher job satisfaction. It turns out, doing causal analysis is a bit like detective work: you pursue various leads and look for evidence of whether each one might be true. If you conclude possibilities 1-4 are all false, then you know possibility 5 must be true.
Randomized control trials (RCTs) are often considered the gold standard of causal research. That’s because in an RCT, it’s not possible that job satisfaction or some confounding factor caused people to participate (Possibilities 1 and 2); instead, the researcher’s randomization process caused some people to join in the program and others to join the control group. Thus, we only need to consider Possibilities 3 and 4 before drawing a causal conclusion from a correlation.
Since RCTs are often impractical or unethical, researcher often resort to other methods (which can be quite complex) in order to deal with possibilities 1-4. Some studies allow us to rule out possibilities 1-4 with a high level of confidence while other studies do little to address these possibilities.
The varying quality of evidence regarding causality is already being reflected in some policy briefs written to summarize the state of evidence, with researchers often distinguishing between causal and correlational studies. This binary distinction is too crude for my taste, since I tend to view confidence about causal relationships as a continuum.
But regardless of the language used, I suspect that going forward, we will see more and more summaries of the evidence taking some care to distinguish among studies depending on how strongly they support a causal interpretation.
Author: Nathan Favero (nathanfavero.com) is an assistant professor in the School of Public Affairs at American University. His research focuses on topic related to public management, education policy, social equity, and research methods.
Twitter: @favero_nate




(1 votes, average: 5.00 out of 5)
Loading...
Thinking Clearly About Causality
The views expressed are those of the author and do not necessarily reflect the views of ASPA as an organization.
By Nathan Favero
October 27, 2019
Even with the benefit of hindsight, it can be hard to sort out the precise effects of a decision.
Maybe I notice an uptick in employee productivity following a goal-setting workshop. But was the uptick really caused by the workshop? Or did the addition of a new colleague around the same time boost everyone’s dedication?
The topic of causality is a natural follow-up to my last column on evidence-based management. After all, social scientists are increasingly focused on trying to improve our understanding of causal relationships as we go about assembling evidence.
My favorite tool for thinking about causality is a simple framework that can be used with any two variables. To illustrate, let’s use the example of a professional development program and its effect on job satisfaction.
Suppose I notice that employees who participate in the program are more satisfied with their jobs. In other words, participation is correlated with satisfaction. There are five different reasons we might find this correlation:
Possibility 1: Reverse-causality. My two variables might be correlated because satisfaction causes participation in the program. Satisfaction with the job may drive people to do more, leading them to sign up for the professional development program. If so, I would likely find that those who had participated in the program were happiest with their jobs, even if the program itself had no effect on job satisfaction.
Possibility 2: A confounding factor. Some third variable (a confounding factor) may drive the correlation between participation and satisfaction. For example, suppose that workloads of employees vary widely, with some employees feeling overworked to the point of frustration. Perhaps only those with a manageable workload (who also enjoy their jobs) feel that they can afford the time required to participate in the professional development program. In this case, employee workload is a key driver of both job satisfaction and participation in the program, causing satisfaction and participation to be correlated with one another.
Possibility 3: Coincidence. The world is full of seemingly-random coincidences, so a non-zero correlation can arise without any real reason or explanation. This problem occurs most often with small samples or with small correlations. If only two employees participated in the professional development program, I shouldn’t read too much into the fact that they’re both especially happy with their jobs. With such a small sample, it could be a coincidence. This concern is linked to the concept of, “Statistical insignificance,” which helps to weed out results based on small samples, when such coincidences are likely.
Possibility 4: Research design problems. This is a somewhat broad category of possibilities but includes things like measurement error or people dropping out of your sample. Suppose that the people who participate in the professional development program are told by the program directors that they’ll later be surveyed, and that the directors will look bad unless participants say they now love their jobs. Results of future attempts to measure job satisfaction may now be contaminated if program participants don’t want to disappoint the program directors. Or suppose that participation in the program helps to clarify for employees whether they are a good fit for the organization, and thus accelerates leaving the organization for employees who are unhappy. Even if the program didn’t alter satisfaction, a survey of program alumni might find only happy employees since the others have already left.
Possibility 5: Causality. If you can rule out the prior four possibilities, then you can pretty safely conclude that participation in the program did indeed cause higher job satisfaction. It turns out, doing causal analysis is a bit like detective work: you pursue various leads and look for evidence of whether each one might be true. If you conclude possibilities 1-4 are all false, then you know possibility 5 must be true.
Randomized control trials (RCTs) are often considered the gold standard of causal research. That’s because in an RCT, it’s not possible that job satisfaction or some confounding factor caused people to participate (Possibilities 1 and 2); instead, the researcher’s randomization process caused some people to join in the program and others to join the control group. Thus, we only need to consider Possibilities 3 and 4 before drawing a causal conclusion from a correlation.
Since RCTs are often impractical or unethical, researcher often resort to other methods (which can be quite complex) in order to deal with possibilities 1-4. Some studies allow us to rule out possibilities 1-4 with a high level of confidence while other studies do little to address these possibilities.
The varying quality of evidence regarding causality is already being reflected in some policy briefs written to summarize the state of evidence, with researchers often distinguishing between causal and correlational studies. This binary distinction is too crude for my taste, since I tend to view confidence about causal relationships as a continuum.
But regardless of the language used, I suspect that going forward, we will see more and more summaries of the evidence taking some care to distinguish among studies depending on how strongly they support a causal interpretation.
Author: Nathan Favero (nathanfavero.com) is an assistant professor in the School of Public Affairs at American University. His research focuses on topic related to public management, education policy, social equity, and research methods.
Twitter: @favero_nate
Follow Us!